Artificial Intelligence (AI) is rapidly transforming industries, offering businesses unparalleled opportunities for growth, efficiency, and innovation. However, to fully capitalize on AI's potential, companies must establish a robust infrastructure that can support the computational demands and data processing requirements of AI technologies. At SiUX Technology, we understand the critical role that a well-structured AI infrastructure plays in achieving business goals. This blog will explore the key components necessary for setting up an infrastructure that effectively leverages AI.
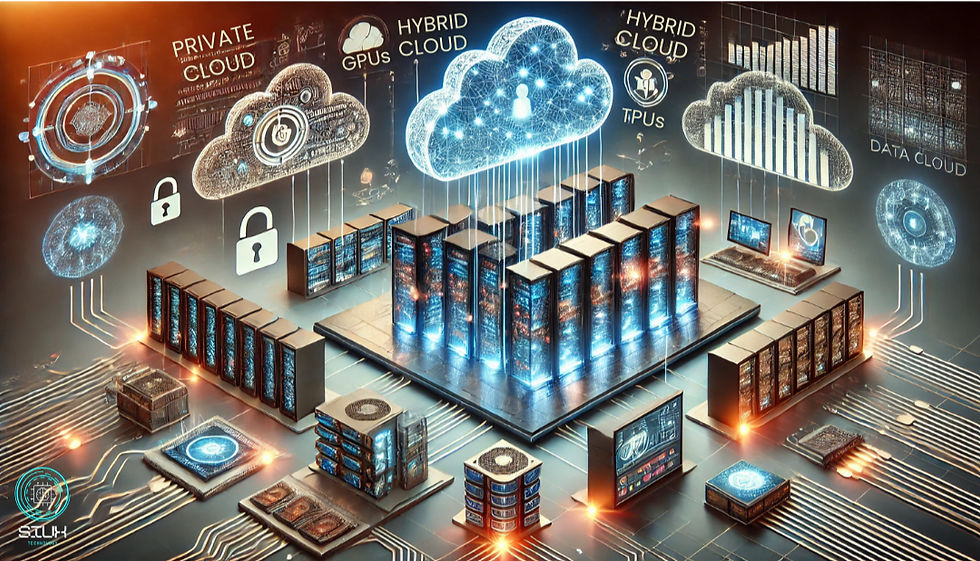
High-Performance Computing (HPC) Resources
AI applications, particularly those involving machine learning and deep learning, require significant computational power. High-Performance Computing (HPC) resources are essential for processing large datasets and running complex algorithms. To build an AI-ready infrastructure, businesses should consider the following:
GPUs and TPUs: Graphics Processing Units (GPUs) and Tensor Processing Units (TPUs) are crucial for accelerating AI computations. Unlike traditional CPUs, GPUs and TPUs are optimized for parallel processing, which is ideal for training AI models. Investing in these specialized hardware components can significantly reduce training times and improve model accuracy.
Scalable Computing Clusters: Businesses should deploy scalable computing clusters that can handle the intensive workloads of AI tasks. These clusters should be designed to scale up or down based on demand, ensuring that resources are utilized efficiently. Cloud-based clusters, such as those offered by major cloud service providers, can provide the flexibility needed to manage fluctuating workloads.
Robust Data Storage and Management Solutions
Data is the lifeblood of AI. To leverage AI effectively, businesses need a robust data storage and management strategy that ensures data is accessible, secure, and well-organized.
Data Lakes and Warehouses: Data lakes and warehouses provide centralized repositories for storing large volumes of structured and unstructured data. Data lakes are particularly useful for AI applications as they allow businesses to store raw data in its native format, making it easier to access and analyze. Data warehouses, on the other hand, are optimized for structured data and are ideal for running complex queries and generating reports.
High-Speed Data Transfer: To support AI workloads, businesses need high-speed data transfer capabilities. This includes high-bandwidth networks that can handle large data transfers between storage systems and computing resources. Implementing a high-speed, low-latency network infrastructure is critical for minimizing bottlenecks and ensuring efficient data processing.
Data Governance and Compliance: Effective data management also involves robust data governance policies. Businesses must ensure that data is handled ethically and complies with relevant regulations, such as GDPR or CCPA. Implementing data encryption, access controls, and regular audits can help maintain data integrity and security.
AI-Optimized Software and Tools
The software stack is as important as the hardware when setting up an AI-ready infrastructure. Businesses should invest in AI-optimized software and tools that facilitate model development, training, and deployment.
Machine Learning Frameworks: Popular machine learning frameworks such as TensorFlow, PyTorch, and scikit-learn provide the tools needed to build and train AI models. These frameworks are continuously updated with new features and optimizations, ensuring compatibility with the latest hardware and software advancements.
AI Development Platforms: Integrated AI development platforms offer a comprehensive suite of tools for building, training, and deploying AI models. Platforms like Microsoft Azure AI, Google AI Platform, and Amazon SageMaker provide end-to-end solutions that simplify the AI development process, allowing businesses to focus on innovation rather than infrastructure management.
MLOps Tools: MLOps (Machine Learning Operations) tools are essential for managing the AI lifecycle, from development to deployment and monitoring. These tools help automate workflows, ensure model reproducibility, and facilitate collaboration between data scientists and engineers. Implementing MLOps practices can streamline the deployment process, reduce errors, and improve overall model performance.
Cloud Infrastructure and Hybrid Solutions
While on-premises infrastructure offers control and customization, many businesses are turning to cloud solutions to meet their AI needs. Cloud infrastructure provides several advantages for AI workloads, including flexibility, scalability, and cost-effectiveness.
Cloud Computing Services: Cloud service providers such as AWS, Google Cloud, and Microsoft Azure offer a range of AI and machine learning services. These services provide businesses with access to powerful computing resources, specialized AI hardware, and pre-built AI models. Leveraging cloud infrastructure allows businesses to scale their AI initiatives quickly without significant upfront investments.
Hybrid Cloud Solutions: For businesses looking to balance control and flexibility, hybrid cloud solutions offer the best of both worlds. Hybrid solutions combine on-premises infrastructure with cloud services, enabling businesses to manage sensitive data in-house while leveraging the scalability and cost benefits of the cloud. This approach also provides greater flexibility in optimizing workloads based on performance and cost considerations.
Reliable Networking and Connectivity
A reliable and high-performance network is essential for AI infrastructure, especially for businesses using cloud-based resources or distributed computing environments.
Low Latency Networks: AI applications, particularly those involving real-time data processing or machine learning training, require low-latency networks to minimize delays and ensure efficient data transfer. Implementing high-speed fiber optic networks, reducing network hops, and optimizing data paths can significantly improve performance.
Secure Connectivity Solutions: Securing the network infrastructure is critical, especially when dealing with sensitive data or deploying AI models in production environments. Virtual Private Networks (VPNs), firewalls, and encryption protocols can help protect data in transit and ensure secure communication between different infrastructure components.
Comprehensive Monitoring and Management Tools
To maintain a robust AI infrastructure, businesses need comprehensive monitoring and management tools that provide real-time insights into system performance, resource utilization, and potential issues.
Infrastructure Monitoring: Tools like Prometheus, Grafana, and Nagios offer real-time monitoring capabilities, allowing businesses to track the health and performance of their AI infrastructure. These tools can help identify bottlenecks, predict failures, and optimize resource allocation, ensuring smooth operation and maximum efficiency.
AI Model Monitoring: Monitoring AI models in production is crucial to ensure they perform as expected and deliver accurate results. Tools like ModelDB and Fiddler provide visibility into model performance, allowing businesses to detect drifts, bias, and other issues early. Continuous monitoring and retraining are essential to maintain model accuracy and relevance.
Conclusion
Building a robust AI infrastructure is essential for businesses looking to leverage the full potential of artificial intelligence. From high-performance computing resources and data management solutions to cloud infrastructure and comprehensive monitoring tools, each component plays a vital role in ensuring AI initiatives' success. At SiUX Technology, we specialize in helping businesses design, implement, and manage AI-ready infrastructure tailored to their unique needs. Whether you're just starting your AI journey or looking to optimize your existing setup, our team of experts is here to guide you every step of the way.
For more information on how SiUX Technology can help you build a robust AI infrastructure, visit our website at www.siuxtechnology.com or reach out, we are here to help.
Comments