A data lake is a centralized repository designed to store vast amounts of raw data in its native format, whether structured, semi-structured, or unstructured. Unlike traditional databases, which require data to be organized and structured before storage, data lakes accept data in its original form, making them highly flexible and scalable.
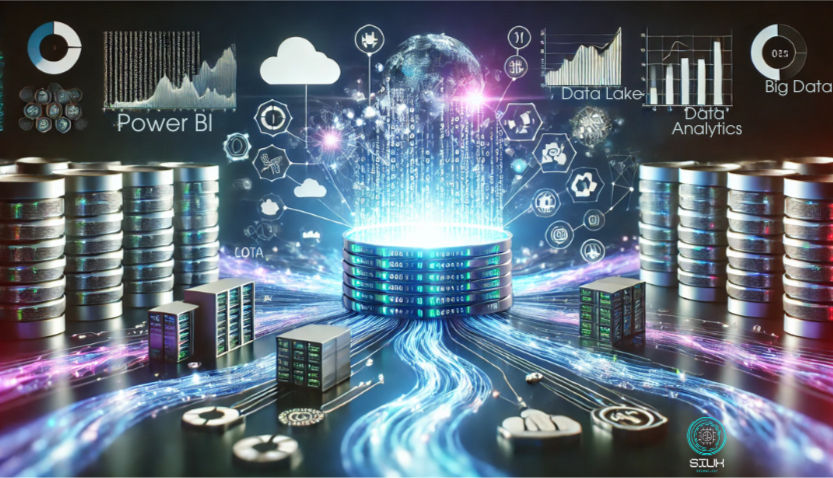
Key features of a data lake include:
Scalability: Data lakes can handle petabytes of data, making them suitable for enterprises managing large-scale data operations.
Diversity: They support a wide range of data types, including text, images, videos, IoT data, and logs.
Flexibility: Data can be stored indefinitely and processed only when needed, reducing upfront costs and complexities.
How Do Data Lakes Differ from Data Warehouses?
While both data lakes and data warehouses store data, their purpose, structure, and usage differ significantly.
Feature | Data Lake | Data Warehouse |
Data Format | Raw, unprocessed data | Processed, structured data |
Data Types | Structured, semi-structured, unstructured | Primarily structured |
Storage Cost | Lower cost due to simple architecture | Higher cost due to specialized hardware |
Users | Data scientists, engineers | Business analysts, executives |
Processing Model | Schema-on-read (structure data when accessed) | Schema-on-write (structure data upon storage) |
Benefits of Data Lakes
1. Scalability and Cost-Effectiveness
Data lakes are built on cost-efficient storage systems such as AWS S3 or Azure Data Lake. They can scale horizontally, allowing organizations to store massive amounts of data without significant investment in hardware.
2. Flexibility
The schema-on-read approach allows businesses to store data in its raw format and process it later when specific needs arise. This flexibility accommodates rapidly changing data requirements.
3. Advanced Analytics
Data lakes enable advanced analytics such as machine learning, real-time processing, and predictive modeling. By integrating tools like Apache Spark, TensorFlow, or cloud-based analytics platforms, organizations can extract deeper insights from their data.
4. Centralized Data Access
With all data stored in one location, organizations can eliminate data silos. This centralized access streamlines data discovery, governance, and collaboration.
Challenges of Data Lakes
Despite their benefits, data lakes come with challenges:
1. Data Governance
Without proper governance, data lakes can become "data swamps," filled with redundant or irrelevant information. Implementing strong metadata management and data quality standards is critical.
2. Complexity
Setting up and managing a data lake requires technical expertise and robust infrastructure. Organizations must invest in skilled personnel and technologies to ensure success.
3. Security Concerns
Storing sensitive data in a centralized repository can pose security risks. Implementing encryption, access controls, and compliance measures is essential to mitigate these risks.
Best Practices for Implementing a Data Lake
1. Start with a Clear Strategy
Define the purpose and goals of your data lake. Identify the types of data it will store and the analytics it will support. A clear strategy ensures alignment with business objectives.
2. Choose the Right Platform
Select a data lake platform that aligns with your scalability, performance, and integration needs. Popular options include AWS, Azure, Google Cloud….
3. Implement Robust Data Governance
Establish policies for data quality, cataloging, and metadata management. Tools like Apache Atlas or Alation can help maintain organization and prevent data swamps.
4. Ensure Security and Compliance
Protect your data lake with encryption, role-based access controls, and monitoring tools. Ensure compliance with regulations like GDPR, HIPAA, or CCPA.
5. Integrate Analytics Tools
To unlock the full potential of your data lake, integrate analytics and visualization tools such as Tableau, Power BI, or custom machine learning models.
Applications of Data Lakes
Data lakes are widely used across industries to drive innovation and efficiency:
Healthcare: Store and analyze patient records, medical images, and IoT data from wearable devices.
Finance: Detect fraud, analyze market trends, and optimize investment strategies.
Retail: Personalize marketing campaigns, forecast demand, and enhance customer experiences.
Manufacturing: Monitor IoT sensor data to predict equipment failures and improve operational efficiency.
As mentioned in our previous blog: data lake and big data are easily interchangeable. To be exact, Big data refers to large amounts of data, while a data lake is a repository for storing that data. Big data is a technology concept, while a data lake is a business concept
Conclusion
Data lakes are revolutionizing data management by providing a scalable, flexible, and cost-effective solution for storing diverse data types. They empower organizations to break down silos, uncover deeper insights, and drive data-driven decision-making. However, successful implementation requires careful planning, robust governance, and the right technology stack.
At SiUX Technology, we help businesses design, implement, and optimize data lakes tailored to their unique needs. Contact us today to learn how we can help your organization harness the power of data lakes and transform your data into actionable insights.
Comments